The digital economy and corporate credit risk: An empirical study based on Chinese new energy enterprises
- College of Economics and Management, Taiyuan University of Technology, Taiyuan, China
The integration of digital technologies is exhibiting an upward trend in Chinese enterprises, and the degree of corporate credit risk is directly proportional to their financial sustainability. Based on panel data of new energy enterprises from 2012 to 2020, this article makes an empirical study on the direct effect, mediating effect, and moderating effect of the digital economy (DE) on the new energy enterprises’ credit risk. It is found that the digital economy could significantly mitigate the credit risk of new energy enterprises by improving total factor productivity and amplifying the potential default cost. When the digital economy affects corporate credit risk, the development of the new energy industry acts as an intermediary, and knowledge spillover acts as a moderator. Furthermore, considering knowledge spillover as the threshold variable, the digital economy has a double-threshold effect. The marginal impact fluctuates from dropping to increasing as the knowledge spillover level increases. As for the region’s heterogeneity, the digital economy has benefited eastern China more than central and western China, possibly due to the differences in economic structure, capital intensity, and policy institutions. In view of these findings, this study provides a reference for China to mitigate corporate credit risk in the digital economy era.
1 Introduction
As one of China’s seven emerging strategic industries, the new energy industry has distinct development advantages. Characterized by low consumption, low emissions, and low energy pollution, the production model of the new energy sector satisfies the fundamental criteria for environmental protection and low-carbon development (Chen, 2011). To achieve the “double carbon” goal, the new energy industry becomes the mainstream direction of future growth for China’s energy industry (Zhang and Qian, 2023). Meanwhile, it pertains to an industry that is capital- and technology-intensive. The industrial chain is extensive and comprises numerous sectors. Other businesses in the chain will then have a more significant promotional effect. Therefore, the degree of new energy industrial development has become an essential indicator of a country’s or region’s level of green innovation. However, China’s new energy industry is still in its initial stages of transformation and is exposed to a variety of risks, including credit risk, which is progressively gaining attention.
In the era of the digital economy, new energy enterprises are presented with the historic opportunity and challenge of incorporating green transformation with economic growth. Some advanced companies have significantly increased green product innovation due to the integration of digital technologies (Cioacă, et al., 2020; Jones and Wynn, 2021). According to some earlier studies, the digital economy may impact new energy firms’ performance through three paths (Zhou et al., 2022). The primary objective is to enhance the effectiveness of resource allocation (Nascimento et al., 2018). Data has evolved into a critical component of manufacturing. Its distinct value and function have increased total factor productivity. By integrating data with diverse production activities, the digital economy reshapes new energy firms’ production and working modes and realizes the intelligent linkage of the supply and service chains (Li and Wang, 2022). For instance, digital trading platforms circumvent the limitations of traditional sales models, allowing new energy enterprises to optimize their production processes and design unique solutions and marketing strategies in response to market demand (Qi and Xiao, 2020). Such a business logic increases the marginal utility of customers and promotes the accurate distribution of production factors (Fu et al., 2020). The second objective is to broaden the innovation-driven impact. Digital technologies encourage resource conservation and green production and help address crucial technology constraints (Heo and Lee, 2019). In this sense, the digital economy can effectively enhance an organization’s capacity for technological innovation and ground-breaking invention. The third objective is to transform new energy industries digitally. The increased usage of digital technologies constantly generates new industrial growth points, providing tremendous force for the long-term development of new energy enterprises (Jabłoński, 2018). Besides, scholars also investigate how the digital economy impacts enterprise business models (Tan et al., 2007), organizational structures (Nuryaman, 2015), governance mechanisms (Gopalakrishnan and Mohapatra, 2020), Etc.
Although the digital economy has empowered enterprises to grow sustainably, issues such as the monopoly of Internet giants, the herding effect, the siphoning impact, and information security exacerbate economic instability and negatively influence the development of enterprises. Therefore, there needs to be more concrete evidence concerning the effect of the digital economy on mitigating corporate credit risk. However, whether the digital economy contributes to reducing corporate credit risk? What is the system that allows it to function? What regional peculiarities does the impact of the digital economy have on the credit risk of new energy enterprises? The responses to these questions serve as a guide for decision-making entities. These are also essential for policy implementation and offer theoretical and empirical considerations for developing China’s digital economy.
The marginal contributions of this paper are as follows: Firstly, this study aims to integrate the digital economy and corporate credit risk into a unified research framework and examine the mechanisms, thereby contributing to the relevant literature. Secondly, this paper uses the Gaussian mixture model and constructs an iterative process to determine the value of the company’s assets. This enhances the accuracy and versatility of the KMV model for calculating the credit risk of new energy companies. Thirdly, this study demonstrates the geographical heterogeneity of the effect of the digital economy. Eastern China has benefited from the digital economy more than central and western China, which may be related to variations in economic structure, capital intensity, and institutional frameworks for policymaking.
The remaining sections of this work are as follows. Section 2 presents a literature review. The hypotheses are proposed in Section 3. Section 4 outlines the empirical methods and the data. Section 5 includes empirical evidence on how the digital economy affects the credit risk of new energy firms. Section 6 contains conclusions and policy implications.
2 Literature review
The digital economy has arisen as a new economic paradigm due to the rapid expansion of the information and communication technology industry. It is also a recent main economic activity after agriculture and industry (Pan et al., 2022). According to the “White Paper on the Development of China’s Digital Economy (2021),” China’s digital economy reached RMB 39.2 trillion in 2020, an increase of 9.7 percent annually. In comparison, GDP growth was only 2.3% in the same year. The digital economy continues to develop swiftly, even though the COVID-19 epidemic has dramatically impacted enterprises’ sustainable development and interrupted the national economy’s smooth operation (Ding, 2020; Ding et al., 2021). Against this background, the adoption of digital technology may assist in overcoming the limitations of crucial technologies, hence accelerating the digital transformation of the new energy business. In the field of R&D, digital carriers create favorable conditions for the rapid circulation and accurate distribution of production factors, which improve production efficiency by shortening production time and reducing the costs of operation and maintenance (do Amaral Burghi and Hirsch, 2020). In terms of transportation, AI technology can be employed to discover the optimal solution for the transit and storage of new energy based on the intelligent algorithms of machine learning (Dong et al., 2020). Machine vision technology may be used to complete dynamic evaluation and real-time monitoring and eliminate the interference caused by exceptional items during energy transportation (Zhou et al., 2016). Regarding marketing, the digital trading platform built using cloud computing and blockchain technology has effectively reduced the information imbalance between supply and demand. Therefore, new energy enterprises are empowered to base personalized solutions on the value orientation of customers (Tang et al., 2021). In terms of supervision, the digital twin technology completes mapping all elements between physical objects and virtual models, thus creating a high-fidelity digital world for the new energy industry. Then, the prediction and extrapolation of industrial activities can be carried out from various perspectives (Liu et al., 2018). The abnormal working cycles can be detected in time by comparing the digital model’s virtual evaluation results with the entity industry’s actual measurement results, guiding the attentive monitoring of the industrial process (Sarkis et al., 2020).
Regarding the evolutionary causes of corporate credit risk, scholars have published a great deal of literature. Enterprises’ internal and external environments have been discussed in numerous ways. External factors such as changes in the macro environment, the evolution of social networks, and policy uncertainty can disrupt the production and operations of businesses and impact their credit risk levels. For instance, the onset of the financial crisis has severely hindered the normal functioning of international markets, diminished global consumer demand, and placed companies in financial distress, significantly increasing the likelihood of credit risk occurring (Lu et al., 2012). Social networks’ impact on enterprises’ solvency is most evident in debt financing and R&D expenditures. The greater the social network, the stronger the financing capacity of the company and the quicker the growth of R&D expenditures (Horton et al., 2012). Moreover, economic policy uncertainty can lead to increased business risks, decreased investment conversion rates, and cash shortages, all of which can hinder a company’s capacity to repay its loans and increase its credit risk (Chodnicka-Jaworska, 2022). From the firm’s internal environment perspective, some academics have investigated the impact of enterprise credit risk based on their own situational factors. Capital structure, equity position, and company size will influence corporate credit risk (Boubakri et al., 2013; Zhang and Zhang, 2016). In addition, promotion incentives can indirectly affect corporate credit risk by controlling the risk appetite of executives (Kini and Williams, 2012).
3 Theoretical hypothesis
3.1 Digital economy and corporate credit risk
In the existing literature, many studies explore corporate credit risk factors from the perspectives of solvency and willingness (Bharadwaj, 2000; Peng and Ye, 2011). This study also adopts these two dimensions to examine the connection between the digital economy and corporate credit risk. Regarding solvency, digital technology has gradually permeated all facets of industrial activity, presenting businesses with a new technology paradigm that has challenged their initial production methods and management structures (Jing and Sun, 2019). The digital economy can improve total factor productivity (TFP) through economies of scale and scope (Wang K K et al., 2020). On the one hand, the digital economy has clear network externalities. When a specific number of consumers is reached, enterprises can cut their average costs through bulk transactions, uniform procurement of raw materials, and dilution of R&D expenses. Subsequently, the profits generated by economies of scale can be distributed (Erdem et al., 2016). On the other hand, the diffusion of digital technology has lowered the information threshold for economic activities, broadening the sales coverage of products and promoting the low-cost development of diverse businesses (Hart and Moore, 1994). The economy of scope provides the industry with diversified products and services that satisfy long-tail demand and adds value to the core business. Besides, from the standpoint of the enterprise management, the collaboration tools provided by digital technology allow data to flow efficiently, securely and completely, thus bridging the digital divide between different departments. In this way, enterprises are activated to eliminate redundant organizational layers, for the construction of a networked and flat organizational structure (Jacobides et al., 2018). When companies are confronted with a complex and ever-changing environment, greater decision-making efficacy can significantly decrease business risks and improve their viability and competitiveness (Adner, 2017). This is conducive to preventing credit risks resulting from poor decisions.
Willingness to pay the debt is the subjective attitude of a firm toward defaulting on a debt service contract while having the financial resources to do so. It is related to the opportunistic behavior of enterprises (Abeler et al., 2014). According to the reputation mechanism theory, a company’s reputation will be proportional to its future earnings. If an adverse event, such as a penalty, damages a company’s reputation, it will leave an indelible imprint that will negatively impact its future profitability. The digital economy has spurred the fast growth of online social media. The information distribution is no longer restricted by time or distance. It not only makes it easier for creditors and regulators to oversee management, but its potent public opinion governance and information transmission capabilities will make it difficult for businesses to conceal bad information and raise the cost of default (Zhu et al., 2020). Consequently, rational enterprises are forced to improve information transparency and fulfill their debt repayment obligations to avoid the spread of negative public opinion. Moreover, solvency can influence an enterprise’s willingness to service debt. Some research suggested that firms with superior solvency pay more attention to their corporate reputation, which means they will keep a perfect image by favorably repaying loans for long-term growth (Gino et al., 2009). Therefore, the digital economy can indirectly raise enterprises’ willingness to service debt by enhancing their solvency (Tadelis, 1999). In conclusion, the following hypothesis is offered in the present study:
Hypothesis 1 (H1):. The digital economy can significantly mitigate the credit risk of new energy companies.
3.2 The moderating role of knowledge spillover
As the driving force behind new energy industrial growth shifts from low-cost factors to high-value-added innovation, knowledge is acknowledged as an efficient supplement to traditional element. Some studies concluded that the Internet has contributed to enriching the dissemination of knowledge and breaking the temporal and spatial boundaries of knowledge diffusion, thus permitting the large-scale circulation of intellectual elements (Baldwin et al., 2011). Through the geographical effect of knowledge spillover, some enterprises with sophisticated digital technologies have, to a certain extent, stimulated other enterprises in the same region to improve the integration and adoption of digital technologies. Therefore, the process of digital transformation is accelerated (Zhao and Li, 2011).
There are two primary manifestations of the moderating effect of knowledge spillover in the connection between the digital economy and the credit risk of new energy firms. First, knowledge spillover has an innovation effect. Under regional innovation systems, external factors and accumulated innovation resources and conditions influence each region’s innovation production (Fu, 2009). As regions are gradually absorbed into labor and capital division networks, knowledge and technology spillover channels are created to facilitate interregional collaboration and exchange. Furthermore, the closer geographic proximity between regions increases the likelihood of regional knowledge spillover (Mao, 2019). During the digitization process, a greater degree of knowledge spillovers is advantageous for decreasing R&D costs, boosting innovation output, and addressing the technical needs of new energy firms. Consequently, knowledge spillover improves organizations’ solvency via innovation-driven benefits, thus lowering corporate credit risk. Second, knowledge spillover promotes the release of the agglomeration effect of the digital economy. As the core intellectual element that determines the competitive advantage of enterprises, tacit knowledge is highly ambient, contextual, and abstract (Hidalgo and Hausman, 2009). It can only be disseminated through face-to-face communication (Spender and Grant, 1996). Then, for the quick, precise, and cost-effective acquisition of new knowledge, enterprises will cluster around sources of knowledge spillovers voluntarily (Aydogan and Lyon, 2004). The innovation and business environment that the digital economy has created, meantime, can encourage companies to innovate more effectively and take advantage of intellectual resources, which is essential for the industrial chain to deepen and grow (Shen et al., 2022). Therefore, the higher the level of knowledge spillover, the greater the likelihood that regional new energy firms would experience the collective positive effect of knowledge spillover and the digital economy (Hong and Fu, 2011). This has played a driving role in reducing the credit risk of new energy companies. In summary, the current investigation proposes the following hypothesis:
Hypothesis 2 (H2):. Knowledge spillover can positively moderate the effect of the digital economy on the credit risk of new energy firms.
3.3 The mediating role of new energy industrial development
The upstream and downstream transmission effect of the enterprise is the linchpin of their development process (Garrido and Okhrati, 2018). The digitization of supply, production, and marketing management necessitates close cooperation between upstream and downstream businesses. Otherwise, the digitization of individual companies may reduce communication and coordination efficiency rather than improve them (Aghion et al., 2015). For instance, the digital transformation of an enterprise’s supply chain management system entails not only an investment in digital software and personnel but also the digital entry and administration of the enterprise’s raw material procurement. If the pertinent raw materials and product information is entered solely by this enterprise, the cost will inevitably be substantially higher (Acemoglu and Restrepo, 2018). Therefore, concurrent development of the industry chain will reduce the friction between upstream and downstream enterprises in digitalization and decline the cost of supply chain management system application for enterprises, which contributes to the improvement of the solvency of new energy businesses, hence reducing corporate credit risk. The framework depicted in Figure 1 can be derived from a summary of the study’s hypotheses regarding the relationship between the digital economy, knowledge spillover, and enterprise credit risk. In summary, the following hypothesis is proposed:
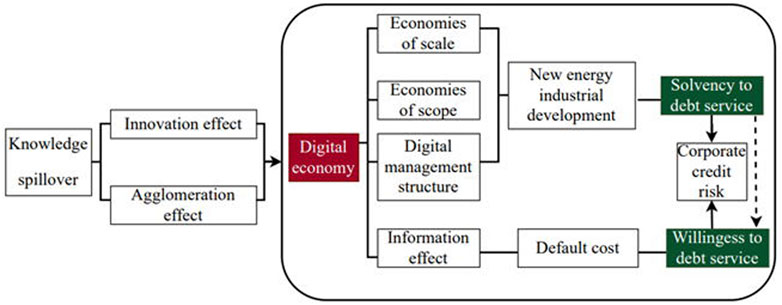
FIGURE 1. The theoretical framework of the digital economy, knowledge spillover, and corporate credit risk.
Hypothesis 3 (H3):. New energy industrial development mediates the link between the digital economy and the credit risk of new energy businesses.
4 Methodology and data
4.1 Variables definition
4.1.1 Dependent variable
Risk management methods are commonly used to evaluate and decrease credit risk (Antràs et al., 2012). This study analyzes corporate credit risk utilizing the KMV model, which is based on the Black and Scholes (1973) and Merton (1974) option pricing models. To verify the stability of the KMV model, Kurbat and Korablev (2002) analyzed the financial data of tens of thousands of US enterprises over a decade. They discovered that the curve of expected default probabilities generated by the KMV model might closely resemble the actual default probabilities. Since enterprise stock prices were considered, Cheng and Chu (2020) demonstrated that the KMV model was more sensitive and accurate than other approaches. In accordance with the Merton model’s assumptions, the equity is equivalent to a European call option with the debt value as the strike price and the asset value as the underlying, which should be assessed using the following equations:
In these formulas, E represents the enterprise’s equity’s market value, V denotes the enterprise’s asset’s market value. D indicates the face value of debt, r reflects the risk-free rate of return, N( ) stands for the cumulative distribution function of normal distribution,
Where
In the theory of option pricing,
Where
In addition, we construct the subsequent iterative process to determine the value of the company’s assets. First, the intermediate variables
4.1.2 Independent variable
The digital economy (
4.1.3 Mediator and moderator
New energy industrial development (
Knowledge spillover (
4.1.4 Control variable
When building a model, the enterprise credit risk is impacted by a few different variables. Therefore, we must ensure that other variables are adequately controlled to conduct an accurate quantitative analysis. Following the research of Guo and Zhu (2021), we use the four indicators as control variables: 1) Enterprise profitability (
4.2 Empirical methods
4.2.1 Fixed-effect model
Panel data is an excellent way to combine cross-section and time series, which not only overcomes the defects of a single method but also can solve the problems of multicollinearity in time series analysis and provide more information, more changes, less collinearity, more degrees of freedom, and improved estimate efficiency. There are usually two types of models for panel data: random effects and fixed effects (Firebaugh et al., 2013). The assumption of zero correlation and the possibility of fluctuation between the observable qualities are two constraints to which the random effect model must adhere (Greene, 2001). Despite this, the fixed-effects method has many advantages. It can lessen the impact of confounding factors on estimation findings, the impact of time-invariant variables and the bias induced by omitted variables (Allison, 1996; Hsiao, 2003). To examine the direct effect of the digital economy on the credit risk of new energy enterprises, we perform the Chow test, LR test, and Hausman test on the panel data. All tests reject the null hypothesis at the 5% level of significance. Therefore, this work builds fixed-effect models for the empirical test. In addition, individual and year-fixed effects are integrated into the model to minimize the impact of differences between individuals and changes over time.
In this formula,
According to the abovementioned findings, there may be moderate mechanisms of action in addition to the direct impact of the digital economy on enterprise credit risk. Improved on Zhang’s (2020) research methods, the specific test steps are as follows:
Where
4.2.2 Panel threshold model
Considering that varying knowledge spillover levels may affect its moderating effect, the panel threshold model proposed by Hansen (1999) is selected to explore whether the threshold effect regulates the independent variables. The followings are the specifics of the single and double threshold models:
In these formulas,
4.3 Data sources and descriptive statistics
Due to the availability and effectiveness of data collection, this study focuses on listed new energy enterprises in China, and the period ranges from 2012 to 2020. In terms of sample selection, we use the cataloged “new energy concept sector” (BK0493) from the “East Money Information Site” to choose cooperative enterprises for research purposes. The financial data of the new energy enterprises used to calculate the distance to default (
This paper processes the data according to the following principles: 1) Enterprises with a listing date after 2012 are eliminated; 2) ST enterprises in the sample are excluded; 3) Enterprises with incomplete data are removed. After the above steps, we obtained 1,008 samples from 112 listed companies. Meanwhile, Tibet, Qinghai, and Xinjiang are not covered since these provinces have few eligible samples. We eventually collected 252 samples in total from 28 provinces.
The descriptive statistics of each variable are shown in Table 3. Specifically,
5 Empirical results analysis
5.1 Basic analysis result
This study adopts the fixed-effect models to examine whether the digital economy helps mitigate the credit risk of new energy enterprises. Table 4 reports the results. Columns 1) and 2) indicate the effects of the digital economy on the credit risk of new energy enterprises. Both the regression coefficients are statistically significant at the 1% level, indicating that new energy enterprise credit risk can be significantly decreased as the digital economy grows, supporting Hypothesis H1.
In Table 4, Columns 3) and 4) show the moderating impact of knowledge spillover. The interaction term between the digital economy and knowledge spillover has significantly positive coefficients. This suggests that knowledge spillover has innovation and agglomeration effects on businesses, moderating the relationship between the digital economy and the credit risk of new energy enterprises. As a result, Hypothesis H2 is confirmed.
The structural equation model is further performed to confirm the mediating effect of the new energy industry development. The estimation deviation is adjusted using the non-parametric bootstrapping method. As shown in Table 5, the coefficient of direct effect is 0.118, and the 95% confidence interval excludes 0 since it is [0.093,0.149]. This indicates the direct effect of the digital economy is significant. The coefficient of mediating effect is 0.023, and the 95% confidence interval excludes 0 with a range of [0.007,0.038]. This indicates that developing new energy industries is a vital mechanism for the digital economy to lower the credit risk of new energy enterprises, hence validating Hypothesis H3.
5.2 Basic analysis by region
Different regions of China have varying degrees of the digital economy. The regional fixed-effect model is adopted to investigate whether there is significant regional heterogeneity. Table 6 reveals that the influence of the eastern region’s digital economy is favorable at 1% significance level. However, the significance of the central and western regions is only 10%. The reason is that the central and western regions may still be in the preliminary phase of digital economic development. In contrast, the convenient geographical conditions of the eastern region have facilitated its growth. Meanwhile, the Chow breakpoint test is employed to verify that the uneven geographical distribution of new energy enterprises does not influence the conclusions. The result demonstrates that the conclusion rejects the null hypothesis regarding the absence of regional heterogeneity at the 5% significance level. In conclusion, the impact of the digital economy on the credit risk of new energy enterprises differs between the two regional samples.
5.3 Threshold effect analysis
This study develops a panel threshold model to test whether knowledge spillover moderates the relationship between the digital economy and the credit risk of new energy enterprises beyond a predetermined threshold. Using knowledge spillover as the threshold variable, Table 7 reports that the digital economy has a double threshold effect on enterprise credit risk, with respective thresholds of 3.381 and 3.819.
Table 8 displays that the impact of the digital economy on the credit risk of new energy enterprises varies according to the level of knowledge spillover. When the knowledge spillover degree is less than 3.381, the digital economy significantly impacts the credit risk of new energy enterprises, and the regression coefficient is 0.266. Although businesses can only innovate using their intellectual capital during this stage, they eventually develop intangible assets and obtain inventive advantages through technological research. When the level of knowledge spillover is between 3.381 and 3.819, the regression coefficient is lower than in the previous period. This change may be because the increased knowledge spillover accelerates the rate of knowledge diffusion and shortens the product life cycle. To keep up with continuously evolving market needs, enterprises must raise their R&D spending and innovation efficiency. As a result, the growing uncertainty produced by the highly competitive innovation environment mitigates the influence of the digital economy on the credit risk of new energy firms. However, the digital economy’s regression coefficient is significantly positive, with a value of 0.292, when the level of knowledge spillover exceeds 3.819. At a high level of knowledge spillover, learning new technologies is less expensive than developing them from scratch. Therefore, enterprises are more likely to convert external intellectual capital and innovative technologies into intrinsic growth drivers. At this phase, knowledge spillover’s cost-saving and efficiency-improving effects will outweigh the uncertainty it creates. Accordingly, the digital economy’s positive effect on reducing corporate credit risk will become increasingly prominent.
5.4 Robustness tests
5.4.1 Endogenous tests
On the hand, there may have endogenous issues that are mutually causative. Therefore, this research employs instrumental variables to address the endogeneity issue. We reference Kim et al. (2014) and use the same yearly average level of the digital economy in other provinces as an instrumental variable (
Table 9 reports the results of endogenous tests. Based on the findings of the first stage in column 1), the instrumental variable is significantly positively linked with the digital economy. As shown by the results of the second stage in column 2), the estimated coefficient of the digital economy (
5.4.2 Replacement of independent variable
Considering that the results of the abovementioned empirical tests might be affected by the selection of indicators, we adopt replacing the independent variable to conduct robustness tests. Initially, government investment in science and technology is viewed as replacing the digital economy. After performing the Hausman test, the fixed-effect model is chosen for regression. According to the results of Tables 10 and 11, even though the independent variable has changed, the digital economy remains significantly advantageous for the credit risk reduction of new energy enterprises. Regarding impact mechanisms, increasing the degree of knowledge spillover and the digital economy is beneficial for mitigating corporate credit risk. And the new energy industrial development is one of its indirect mechanisms for lowering enterprise credit risk. In conclusion, the results of this study’s fixed-effect model are unaffected by alternative measures of the variables.
5.4.3 Replacement of threshold variable
The logarithm of the regional book collection is then selected as the threshold variable substitutable for knowledge spillover. According to the robustness test results of Table 12, the digital economy’s coefficient varies across distinct phases. As the knowledge spillover level increases, the digital economy’s effect initially decreases and subsequently grows. When the degree of knowledge spillover is high, the digital economy plays the most prominent role in reducing enterprise credit risk, which is consistent with the previous empirical results. Therefore, the results of the threshold regression model are robust.
6 Conclusion and policy implications
The new energy has been a booming industry in recent years and will affect future energy usage. However, there are many uncertainties in its development. Combining the growth of the digital economy with an assessment of the credit risk of new energy enterprises is a novel and distinctive perspective of this paper. We used the KMV model, the fixed-effect model, and the PTR methods and drew the following conclusions:
1) Digital economy could mitigate the credit risk of new energy enterprises in three ways: Firstly, the digital economy increases total factor productivity (TFP) by reshaping corporate processes and bolstering financial stability. Secondly, the potential cost of default is exacerbated by the communication effect of the digital economy, which works as an intangible disciplinary control to improve firms’ willingness to repay their debts. Thirdly, new energy industrial development plays an intermediary role, suggesting that the digital economy indirectly affects the credit risk of new energy enterprises through this intermediary factor.
2) In addition, when knowledge spillover is acted as the threshold variable, the digital economy exhibits a double-threshold effect on the corporate credit risk. As the level of knowledge spillover rises, the marginal impact fluctuates from decreasing to growing. Furthermore, when knowledge spillover is at high levels, especially across the threshold value of 13.029, the effect of the digital economy on reducing new energy enterprises’ credit risk is particularly evident.
3) Regional heterogeneity tests reveal that the eastern region has a more favorable influence on enterprise credit risk than the central and western regions. In other words, its positive impact has been more pronounced in China’s eastern provinces than in the central and western regions. The causes could be related to differences in economic location and resource abundance.
To mitigate the credit risk of new energy firms, this research provides the following policy implications:
1) Make the digital economy a “sharp weapon” to reduce corporate credit risk. To achieve this objective, government agencies need to stimulate the growth of the new energy industrial sector by promoting digital construction and integrating digital technology into the new energy industry. Meanwhile, a specialized and competitive integration mechanism based on the production processes, business patterns, and management models of new energy businesses should be developed to enable their digital transformation.
2) Given knowledge spillover’s innovation and agglomeration effects, the government should focus on improving knowledge spillover and regional intellectual capital. Specifically, some innovation participants could be attracted by increasing investment in science and technology, creating an environment encouraging innovation, and establishing platforms for industry-university-research cooperation. These government practices effectively enhance the positive role of the digital economy and reduce corporate credit risk by improving regional knowledge diffusion.
3) China’s regional economy needs to be more balanced since there are significant inequalities in talent, capital, and technology between the eastern and western regions. With the opportunity created by the digital economy, the central and western regions can combine their substantial energy resource advantages to develop the new energy sector. Considering the impact of the digital economy on the credit risk of new energy enterprises, the central and western regions should enhance the government’s public function and guiding role, strengthen the coordinated development of new infrastructure across regions, and accelerate the commercial application of digital technologies.
Despite providing empirical evidence for developing the digital economy and new energy, this study has several limitations. The first limitation is the availability of data. In this research, the data spans only 9 years, and some of the data are only at the provincial level, which lacks representativeness at a broader scale. In future research, a more extended period and additional data levels should be used to test the validity of the results. Second, the impact mechanisms require extensive research and documentation to provide more actionable implementation advice. In particular, the future study should concentrate on refining the selection of the digital economy index to account for regional growth patterns. Thirdly, China’s digital economy is undergoing dynamic and rapid development. The direction of future research should include some factors related to this dynamic digital transformation to shed light on the intricate relationship between corporate credit risk and the growth of the digital economy.
Data availability statement
The original contributions presented in the study are included in the article/supplementary material, further inquiries can be directed to the corresponding author.
Author contributions
Conceptualization, MS; methodology, YD; software, YD; resources, YC; writing-original draft preparation, YD; writing-review and editing, MS, YD, and YC; supervision, MS; validation, MS; project administration, MS and YC; funding support, MS. All authors have read and agreed to the published version of the manuscript.
Funding
This research is a part of the research project funded by the National Social Science Foundation (Grant No. 21BJY125). This work was supported by the National Social Science Fund of China [21BJY125].
Conflict of interest
The authors declare that the research was conducted in the absence of any commercial or financial relationships that could be construed as a potential conflict of interest.
Publisher’s note
All claims expressed in this article are solely those of the authors and do not necessarily represent those of their affiliated organizations, or those of the publisher, the editors and the reviewers. Any product that may be evaluated in this article, or claim that may be made by its manufacturer, is not guaranteed or endorsed by the publisher.
References
Abeler, J., Becker, A., and Falk, A. (2014). Representative evidence on lying costs. J. Public Econ. 113, 96–104. doi:10.1016/j.jpubeco.2014.01.005
Acemoglu, D., and Restrepo, P. (2018). Artificial intelligence, automation, and work. America: NBER working paper.
Adner, R. (2017). Ecosystem as structure: An actionable construct for strategy. J. Manag. 43, 39–58. doi:10.1177/0149206316678451
Aghion, P., Cai, J., Dewatripont, M., Du, L., Harrison, A., and Legros, P. (2015). Industrial policy and competition. Am. Econ. J. Macroecon. 7, 1–32. doi:10.1257/mac.20120103
Allison, P. D. (1996). Fixed-effects partial likelihood for repeated events. Sociol. Methods Res. 25, 207–222. doi:10.1177/0049124196025002003
Antràs, P., Chor, D., Fally, T., and Hillberry, R. (2012). Measuring the upstreamness of production and trade flows. Am. Econ. Rev. 102, 412–416. doi:10.1257/aer.102.3.412
Aydogan, N., and Lyon, T. P. (2004). Spatial proximity and complementarities in the trading of tacit knowledge. Int. J. Industrial Organ. 22, 1115–1135. doi:10.1016/j.ijindorg.2004.09.003
Baldwin, R., Forslid, R., Martin, P., Ottaviano, G., and Robert-Nicoud, F. (2011). “Economic geography and public policy,” in Economic geography and public policy (Beijing, China: Princeton University Press).
Bharadwaj, A. S. (2000). A resource-based perspective on information technology capability and firm performance: An empirical investigation. MIS Q. 24, 169–196. doi:10.2307/3250983
Black, F., and Scholes, M. (1973). The pricing of options and corporate liabilities. J. political Econ. 81, 637–654. doi:10.1086/260062
Boubakri, N., Cosset, J. C., and Saffar, W. (2013). The role of state and foreign owners in corporate risk-taking: Evidence from privatization. J. financial Econ. 108, 641–658. doi:10.1016/j.jfineco.2012.12.007
Chen, J. (2011). China's experiment on the differential electricity pricing policy and the struggle for energy conservation. Energy Policy 39, 5076–5085. doi:10.1016/j.enpol.2011.06.006
Cheng, X. Y., and Chu, X. F. (2020). Credit risk of GEM listed companies based on KMV model. J. Huazhong Univ. Sci. Technol. Med. Sci. 38, 51–57. doi:10.15926/j.cnki.hkdsk.2020.02.008
Chihiro, W., Kashif, N., Yuji, T., and Pekka, N. (2018). Measuring GDP in the digital economy: Increasing dependence on uncaptured GDP. Technol. Forecast. Soc. change 37, 286. doi:10.1016/j.techfore.2018.07.053
Chodnicka-Jaworska, P. (2022). Environmental, social, and governance impact on energy sector default risk—long-term issuer credit ratings perspective. Front. Energy Res. 457, 1. doi:10.3389/fenrg.2022.817679
Cioacă, S. I., Cristache, S. E., Vuță, M., Marin, E., and Vuță, M. (2020). Assessing the impact of ICT sector on sustainable development in the European Union: An empirical analysis using panel data. Sustainability 12, 592. doi:10.3390/su12020592
Ding, C., Liu, C., Zheng, C., and Li, F. (2021). Digital economy, technological innovation and high-quality economic development: Based on spatial effect and mediation effect. Sustainability 2021, 14. doi:10.3390/su14010216
Ding, Z. (2020). Research on the mechanism of digital economy driving high-quality economic development: A theoretical analysis framework. Mod. Econ. Res. 1, 85–92. doi:10.13891/j.cnki.mer.2020.01.011
do Amaral Burghi, A. C., and Hirsch, T. (2020). Pitz-Paal, R. Artificial learning dispatch planning for flexible renewable-energy systems. Energies 13, 1517. doi:10.3390/en13061517
Dong, L., Sun, D., Han, G., Li, X., Hu, Q., and Shu, L. (2020). Velocity-free localization of autonomous driverless vehicles in underground intelligent mines. IEEE Trans. Veh. Technol. 69, 9292–9303. doi:10.1109/tvt.2020.2970842
Erdem, T., Keller, K. L., Kuksov, D., and Pieters, R. (2016). Understanding branding in a digitally empowered world. Int. J. Res. Mark. 33, 3–10. doi:10.1016/j.ijresmar.2015.12.003
Firebaugh, G., Warner, C., and Massoglia, M. (2013). “Fixed effects, random effects, and hybrid models for causal analysis,” in Handbook of causal analysis for social research. Editor S. L. Morgan (Dordrecht, The Netherlands: Springer), 113–132.
Fu, C., Zhang, S. Q., and Chao, K. H. (2020). Energy management of a power system for economic load dispatch using the artificial intelligent algorithm. Electronics 9, 108. doi:10.3390/electronics9010108
Fu, M. (2009). Geographical distance and technological spillover effects: A spatial econometric explanation of technological and economic agglomeration phenomena. China Econ. Q. 8, 1549–1566. doi:10.4236/me.2016.72015
Garrido, J, and Okhrati, R (2018). Desirable portfolios in fixed income markets: Application to credit risk premiums. Risks 6, 23. doi:10.3390/risks6010023
Gino, F., Ayal, S., and Ariely, D. (2009). Contagion and differentiation in unethical behavior: The effect of one bad apple on the barrel. Psychol. Sci. 20, 393–398. doi:10.1111/j.1467-9280.2009.02306.x
Gopalakrishnan, B., and Mohapatra, S. (2020). Insolvency regimes and firms' default risk under economic uncertainty and shocks. Econ. Model. 91, 180–197. doi:10.1016/j.econmod.2020.06.005
Greene, W. (2001). Estimating econometric models with fixed effects. Leonard N: New York University.
Guo, J. T., and Zhu, Y. X. (2021). The impact of digital economy on corporate credit risks. J. Shenzhen Univ. Humanit. Soc. Sci. 38, 69–80.
Hansen, B. E. (1999). Threshold effects in non-dynamic panels: Estimation, testing, and inference. J. Econ. 93, 345–368. doi:10.1016/s0304-4076(99)00025-1
Hart, O., and Moore, J. (1994). A theory of debt based on the inalienability of human capital. Soc. Sci. Electron. Publ. 109, 841–879. doi:10.2307/2118350
Heo, P. S., and Lee, D. H. (2019). Evolution of the linkage structure of ICT industry and its role in the economic system: The case of korea. Inf. Technol. Dev. 25, 424–454. doi:10.1080/02681102.2018.1470486
Hidalgo, C. A., and Hausmann, R. (2009). The building blocks of economic complexity. Proc. Natl. Acad. Sci. 106, 10570–10575. doi:10.1073/pnas.0900943106
Hong, J., and Fu, S. (2011). Information and communication technologies and the geographical concentration of manufacturing industries: Evidence from China. Urban Stud. 48, 2339–2354. doi:10.1177/0042098010388956
Horton, J., Millo, Y., and Serafeim, G. (2012). Resources or power? Implications of social networks on compensation and firm performance. J. Bus. Finance Account. 39, 399–426. doi:10.1111/j.1468-5957.2011.02276.x
Jabłoński, M. (2018). Value migration to the sustainable business models of digital economy companies on the capital market. Sustainability 10, 3113. doi:10.3390/su10093113
Jacobides, M. G., Cennamo, C., and Gawer, A. (2018). Towards a theory of ecosystems. Strategic Manag. J. 39, 2255–2276. doi:10.1002/smj.2904
Jing, W. J., and Sun, B. W. (2019). Digital economy promotes high-quality economic development: A theoretical analysis framework. Economist 2, 66–73. doi:10.16158/j.cnki.51-1312/f.2019.02.008
Jones, P., and Wynn, M. (2021). The leading digital technology companies and their approach to sustainable development. Sustainability 13, 6612. doi:10.3390/su13126612
Kim, Y., Li, H., and Li, S. (2014). Corporate social responsibility and stock price crash risk. J. Bank. Finance 43, 1–13. doi:10.1016/j.jbankfin.2014.02.013
Kini, O., and Williams, R. (2012). Tournament incentives, firm risk, and corporate policies. J. Financial Econ. 103, 350–376. doi:10.1016/j.jfineco.2011.09.005
Kurbat, M., and Korablev, I. (2002). Methodology for testing the level of the EDF credit measure. Hyderabad: KMV Corporation.
Li, C., and Wang, S. (2022). Digital optimization, green R&D collaboration, and green technological innovation in manufacturing enterprises. Sustainability 14, 12106. doi:10.3390/su141912106
Liu, S. A., and Chai, C. L. (2011). Analysis of knowledge spillover effect from the perspective of manufacturing agglomeration and R&D investment. Sci. Technol. Forum 07, 32–37+57. doi:10.13580/j.cnki.fstc.2011.07.015
Liu, D. T., Guo, K., Wang, B. K., and Peng, Y. (2018). Summary and perspective survey on digital twin technology. Chin. J. Sci. Instrum. 39, 1–10. doi:10.19650/j.cnki.cjsi.J1804099
Lu, Z., Zhu, J., and Zhang, W. (2012). Bank discrimination, holding bank ownership, and economic consequences: Evidence from China. J. Bank. Finance 36, 341–354. doi:10.1016/j.jbankfin.2011.07.012
Mao, Q. L. (2019). Time-space compression, spatial knowledge spillovers, and industrial upgrading. Stud. Sci. Sci. 37, 422–435. doi:10.16192/j.cnki.1003-2053.2019.03.006
Merton, R. C. (1974). On the pricing of corporate debt: The risk structure of interest rates. J. finance 29, 449–470. doi:10.2307/2978814
Nascimento, D. L. M., Alencastro, V., Quelhas, O. L. G., Caiado, R. G. G., Garza-Reyes, J. A., Rocha-Lona, L., et al. (2018). Exploring industry 4.0 technologies to enable circular economy practices in a manufacturing context: A business model proposal. J. Manuf. Technol. Manag. 30, 607–627. doi:10.1108/jmtm-03-2018-0071
Nuryaman, N. (2015). The influence of intellectual capital on the firm’s value with the financial performance as intervening variable. Procedia–Social Behav. Sci. 211, 292–298. doi:10.1016/j.sbspro.2015.11.037
Pan, W., Xie, T., Wang, Z., and Ma, L. (2022). Digital economy: An innovation driver for total factor productivity. J. Bus. Res. 139, 303–311. doi:10.1016/j.jbusres.2021.09.061
Peng, H. F., and Ye, Y. G. (2011). Loan-pricing model based on repayment ability and repayment willingness. Chin. J. Manag. Sci. 19, 40–47. doi:10.16381/j.cnki.issn1003-207x.2011.06.004
Qi, Y. D., and Xiao, X. (2020). Transformation of enterprise management in the era of digital economy. J. Manag. World 36, 135–152. doi:10.19744/j.cnki.11-1235/f.2020.0091
Sarkis, J., Kouhizadeh, M., and Zhu, Q. S. (2020). Digitalization and the greening of supply chains. United Kingdom: Industrial Management & Data Systems.
Shen, M. H., Tan, W. J., and Chen, Z. Y. (2022). The impact of digital economy development on enterprise innovation: Empirical evidence from Chinese listed companies. South China Finance 02, 30–44.
Spender, J. C., and Grant, R. M. (1996). Knowledge and the firm: Overview. Strategic Manag. J. 17, 5–9. doi:10.1002/smj.4250171103
Tadelis, S. (1999). What's in a name? Reputation as a tradeable asset. Am. Econ. Rev. 89, 548–563. doi:10.1257/aer.89.3.548
Tan, H. P., Plowman, D., and Hancock, P. (2007). Intellectual capital and financial returns of companies. J. Intellect. Cap. 8, 76–95. doi:10.1108/14691930710715079
Tang, X. Y., Liang, Y., and Sun, B. (2021). Application prospect of digital twinning technology in regional multi -energy systems. South. Power Syst. Technol. 15, 104–114. doi:10.13648/j.cnki.issn1674-0629.2021.05.013
Tapscott, D. (1996). The digital economy promise and peril in the age of networked intelligence. New York: MacGraw Hill, 1987–1992.
Wang, K. K., Wu, G. B., and Zhang, G. J. (2020). Has the development of the digital economy improved production efficiency? Economist 10, 24–34. doi:10.16158/j.cnki.51-1312/f.2020.10.004
Zhang, C. (2020). Clans, entrepreneurship, and development of the private sector in China. J. Comp. Econ. 48, 100–123. doi:10.1016/j.jce.2019.08.008
Zhang, H. H., and Zhang, L. Y. (2016). Ownership, tournament incentive and risk-taking. Econ. Manag. 38, 110–121. doi:10.19616/j.cnki.bmj.2016.05.011
Zhang, J., and Qian, F. (2023). Digital economy enables common prosperity: Analysis of mediating and moderating effects based on green finance and environmental pollution. Front. Energy Res. 10, 1991. doi:10.3389/fenrg.2022.1080230
Zhang, X. L., and Jiao, Y. X. (2017). A preliminary study of China’s digital economy development index and its application. Zhejiang Soc. Sci. 04, 32–40+157. doi:10.14167/j.zjss.2017.04.005
Zhao, H., and Li, Y. (2011). A technology innovation model of leading enterprise dominant cluster— considering the distance decay effect of technological spillover. Sci. Res. Manag. 32, 157–164. doi:10.19571/j.cnki.1000-2995.2011.06.020
Zhou, K. L., Yang, S. L., and Shao, Z. (2016). Energy Internet: The business perspective. Appl. Energy 178, 212–222. doi:10.1016/j.apenergy.2016.06.052
Zhou, Z., Liu, W., Cheng, P., and Li, Z. (2022). The impact of the digital economy on enterprise sustainable development and its spatial-temporal evolution: An empirical analysis based on urban panel data in China. Sustainability 14, 11948. doi:10.3390/su141911948
Keywords: digital economy, credit risk management, knowledge spillover, new energy enterprises, KMV model
Citation: Su M, Duan Y and Cui Y (2023) The digital economy and corporate credit risk: An empirical study based on Chinese new energy enterprises. Front. Energy Res. 11:1141793. doi: 10.3389/fenrg.2023.1141793
Received: 10 January 2023; Accepted: 13 March 2023;
Published: 24 March 2023.
Edited by:
Wen-Tsao Pan, Hwa Hsia University of Technology, TaiwanReviewed by:
Crenguța-Ileana Sinisi, University of Pitești, RomaniaFeng Hu, Zhejiang Gongshang University, China
Copyright © 2023 Su, Duan and Cui. This is an open-access article distributed under the terms of the Creative Commons Attribution License (CC BY). The use, distribution or reproduction in other forums is permitted, provided the original author(s) and the copyright owner(s) are credited and that the original publication in this journal is cited, in accordance with accepted academic practice. No use, distribution or reproduction is permitted which does not comply with these terms.
*Correspondence: Min Su, sumin@tyut.edu.cn; Yu Duan, duanyu0997@link.tyut.edu.cn
†These authors have contributed equally to this work and share first authorship